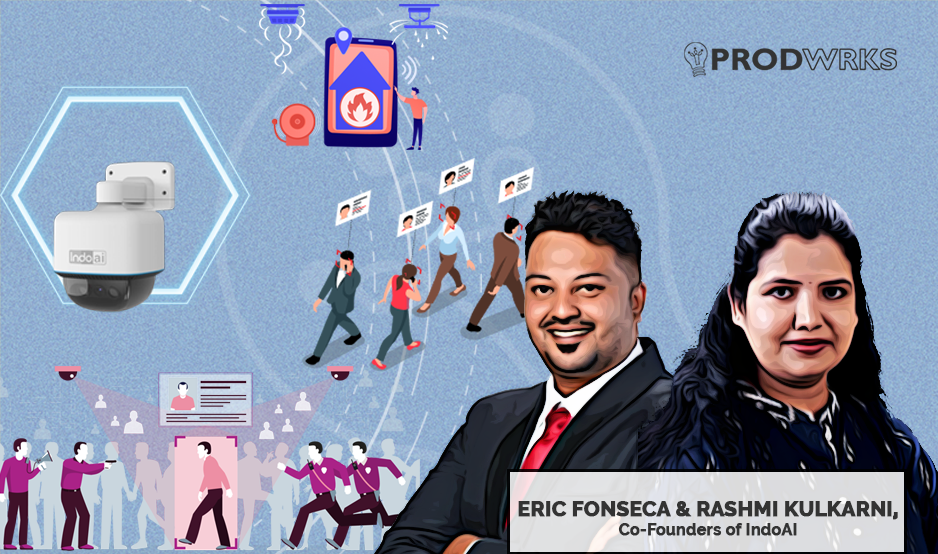
What if CCTVs could think? Not just record, but truly understand what it sees—spot anomalies, detect threats, and offer insights in real-time. That’s the promise delivered by IndoAI’s founders, Rashmi Kulkarni and Eric Fonseca.
With their EdgeAI-enabled cameras and a unique “Appization” business model, IndoAI is reimagining surveillance in 2024 by turning cameras into intelligent, adaptable tools that transform data into action.
Rashmi explains, “At the core of IndoAI’s offering is the Edge AI Camera. It’s a next-generation smart device built on the NVIDIA Jetson Nano platform, capable of processing complex data locally without the need for cloud infrastructure.”
“Users can browse, select, and install AI models tailored to their specific industry needs in IndoAI cameras, just like how we would download and install apps to our smartphones,” says Rashmi.
Currently, the IndoAI marketplace offers four powerful AI models, each designed to address critical needs across industries:
1. Face Recognition: Employed for security, retail, healthcare, and education. In security, face recognition enhances access control for restricted areas. Retail stores can use it for VIP customer recognition or monitoring authorized personnel, while healthcare facilities utilize it for patient and staff identification. Schools and colleges benefit from automated attendance systems using facial recognition.
2. Vehicle Number Plate Detection: Used in transportation, parking management, residential complexes, and law enforcement. This model is essential for monitoring vehicle access in gated communities, tracking unauthorized vehicles, and managing entry/exit in high-security areas. In parking management, it helps streamline operations by identifying vehicles instantly, while law enforcement can use it to track suspect vehicles.
3. Fire & Smoke Detection: Used in manufacturing, warehousing, retail, and residential buildings. This model enhances safety by detecting early signs of fire or smoke, especially useful in manufacturing plants, warehouses, and retail stores where fire hazards are present. In residential buildings, it adds a layer of proactive fire monitoring, helping to prevent potential disasters.
4. Gesture Recognition (Shoplifting Detection): Tailored for retail industry, this model identifies suspicious gestures associated with shoplifting, enabling store personnel to respond swiftly. The model can also be adapted for use in other public or commercial spaces where theft prevention is critical.
When used together, all four models deliver even more comprehensive solutions.
“For example, a retail store could use face recognition for staff access, vehicle number plate detection for VIP or restricted parking, shoplifting detection to prevent theft, and fire & smoke detection to ensure in-store safety,” says Rashmi.
Curious to know more, we interviewed Rashmi, who delved into the limitations of traditional CCTV systems, how IndoAI’s cameras deliver instant insights accessible for businesses across industries, the importance of local data processing for privacy, and why the future of surveillance is all about thinking on the edge.
This interview has been edited for clarity and length
Q1. Traditional CCTV surveillance systems have been around for quite some time, but they come with certain limitations. What are the key drawbacks of these systems that IndoAI’s Edge AI cameras address?
Rashmi: Traditional CCTV systems have long been the standard for surveillance, but they come with several limitations. For starters, standard CCTV cameras merely record footage, requiring manual review to detect incidents—whereas IndoAI’s Edge AI cameras process data on the spot, recognizing faces, identifying objects, and instantly alerting to unusual behavior. Traditional systems also rely on constant streaming to centralized servers, consuming significant bandwidth and storage. In contrast, IndoAI’s cameras process data locally, reducing bandwidth use and minimizing storage demands.
Additionally, response times can be slow with traditional setups, as footage needs review before action is taken, while IndoAI cameras deliver real-time alerts that enable faster, proactive decisions. Another drawback of basic CCTV systems is their limited customization. IndoAI’s cameras, however, support ‘appization’, allowing them to be customized for applications beyond security—such as customer behavior analysis or environmental monitoring.
Lastly, traditional systems lack automation, whereas IndoAI’s AI-driven cameras not only capture video but also learn and adapt, providing ongoing insights and adjusting based on observed patterns. IndoAI’s Edge AI cameras redefine what’s possible, making surveillance more efficient, intelligent, and adaptable.
Q2. Please walk us through the design and core technology behind the IndoAI Edge AI cameras.
Rashmi: IndoAI’s Edge AI cameras are designed to deliver intelligent, real-time analytics directly at the source, removing the need for constant cloud connectivity. At their core, these cameras are powered by the NVIDIA Jetson Nano platform, known for its strong AI processing capabilities, which enable them to perform complex computations on-device, ensuring swift responses and minimal latency.
Unique features include on-device processing, which reduces dependency on external servers and enhances data privacy, customizable AI models through the Appization platform, and broad application versatility, supporting facial recognition, object detection, and environmental monitoring across sectors.
Additionally, the cameras are energy-efficient, thanks to NVIDIA’s technology, which balances high performance with low power consumption, and they prioritize data security by processing information locally, reducing the risk of data breaches.
A key feature that sets IndoAI’s cameras apart is “Appization,” allowing users to customize and deploy AI models tailored to specific applications, much like installing apps on a smartphone. This flexibility makes the cameras adaptable across various industries, from security and surveillance to retail and healthcare.
Q3. The “Appization” model sounds like an interesting concept. Can you elaborate how businesses and developers use it to deploy and customize AI applications?
Rashmi: Just as the Android and iOS mobile ecosystems transformed feature phones by allowing users to download apps for everything from navigation to gaming, Appization allows users to install specialized AI applications directly on IndoAI cameras. This turns the camera into a highly customizable, multifunctional tool tailored to diverse needs—whether it’s for security, retail analytics, or healthcare monitoring.
For third-party developers, Appization opens up a new market. Developers can create AI applications specifically for IndoAI cameras, knowing there’s a ready user base looking to expand their camera’s capabilities. Just as app developers for mobile devices benefit from the popularity of the platforms, developers here can design niche applications, such as facial recognition for offices or vehicle tracking for parking lots, and deliver them directly to customers via the Appization platform.
Camera customers, including businesses in retail, healthcare, or public safety, gain a unique advantage. Rather than buying separate equipment for different tasks, they can update their IndoAI cameras with new applications, saving on both cost and installation time. If a retail store wants to switch from security monitoring to customer behavior analysis, they just need to download the relevant app, transforming the camera’s function instantly without needing new hardware. This flexibility is a huge leap over traditional systems, which are limited to a single-purpose setup.
For IndoAI, Appization is a win because it allows the brand to continually grow its ecosystem and appeal. Much like how app stores have boosted loyalty to Android and iOS, the IndoAI ecosystem of applications will encourage customers to remain within the IndoAI platform. Customers will have a reason to keep their cameras updated, increasing retention, and will also benefit from regular innovation by third-party developers. In short, Appization builds a flexible, sustainable AI camera ecosystem that benefits everyone involved—developers, customers, and IndoAI itself.
Q4. How has the response been from the developer community? How do you plan to grow and the community with resources, tools, documentation, etc.?
Rashmi: Developers have responded positively to the flexibility IndoAI cameras provide for deploying custom AI models, recognizing the potential for varied applications across industries. However, they face challenges like ensuring model accuracy and compatibility on edge devices with limited resources. IndoAI is dedicated to addressing these challenges by offering a robust support framework, including detailed documentation, user-friendly development kits, and comprehensive testing tools.
To assist developers further, we’re introducing tools specifically designed for AI model testing, which allow developers to assess their models’ performance, reduce hallucinations, and identify areas where models may need refinement or pruning to run efficiently on minimal hardware specifications.
Our testing suite will simulate real-world conditions to help developers fine-tune their models, making them more responsive and lightweight without sacrificing accuracy. Additionally, we’re prioritizing model optimization techniques, such as quantization and pruning, to ensure that AI applications can deliver high performance on IndoAI’s edge cameras. Through these resources, we aim to build a strong developer community that has the tools needed to innovate confidently, knowing that their models can adapt to the demands of edge AI applications.
Q5. Edge computing is a significant shift from traditional cloud-based computing. What were the primary technical obstacles you encountered in ensuring real-time data processing and decision-making without cloud dependency?
Rashmi: Shifting IndoAI cameras from traditional cloud-based AI solutions to edge computing presented several technical challenges to achieve real-time data processing and decision-making without cloud dependency. First, edge devices like cameras operate with limited processing power and memory compared to cloud servers, so we needed to optimize AI models extensively. Using techniques like quantization and pruning, we reduced model size and complexity to enable efficient, accurate AI inference directly on the device.
Energy efficiency was another significant challenge—running continuous AI computations on edge devices can quickly drain power. To address this, we designed power-efficient algorithms and leveraged hardware accelerators to keep energy consumption low without compromising speed. Latency was also a major focus, as real-time responses are essential for applications like security monitoring. By streamlining data pipelines and minimizing network dependency, we ensured that the camera could instantly process data and detect actions without delays.
Additionally, while local processing enhances data privacy, it requires strong on-device security. We embedded encryption protocols and strict access controls to protect sensitive information and ensure data security.
Lastly, with edge environments encountering a variety of real-world scenarios, model adaptability was crucial. We engineered flexible models capable of handling diverse use cases, such as differentiating between normal customer browsing and suspicious behavior like shoplifting, without relying on the cloud. Transitioning to edge computing required careful balancing of performance, efficiency, and security, but it allows IndoAI cameras to deliver reliable, real-time insights at the edge.
Q6. How does the camera’s real-time processing and on-device decision-making improve operational efficiency and outcomes?
Rashmi: In industries such as security and industrial automation, IndoAI Edge cameras with real-time processing and on-device decision-making offer a substantial boost in operational efficiency and outcomes. By analyzing data directly at the source, IndoAI Edge cameras reduce latency, enabling immediate responses to critical events without depending on cloud-based processing.
This immediacy is vital for applications like intrusion detection, where quick action can prevent security breaches, and in industrial settings, where real-time monitoring of machinery helps detect anomalies early, preventing costly downtime. Additionally, on-device processing with IndoAI Edge cameras minimizes bandwidth usage and enhances data privacy, as sensitive information doesn’t need to be transmitted over networks.
This localized intelligence allows for more scalable, responsive, and reliable systems, leading to improved safety, productivity, and overall operational effectiveness.
Q7. Real-time AI processing can sometimes cause biases. What kind of biases did you encounter? For example, in a shopping mall, how does the IndoAI camera differentiate between complex scenarios such as theft versus normal shopping behavior?
Rashmi: In real-time AI processing, biases can emerge from training data that doesn’t fully represent the diversity of real-world scenarios, leading to misinterpretations in complex behaviors. For instance, in security applications like theft detection, distinguishing between normal shopping activities and suspicious behavior is challenging. IndoAI cameras address these potential biases by employing advanced machine learning algorithms trained on extensive and diverse datasets, encompassing a wide range of behaviors and contexts.
But we are very far from acceptable limits as of now, but we are learning fast. This comprehensive training enables the system to accurately differentiate between benign actions, such as a customer browsing items, and genuine threats, like shoplifting. Additionally, the cameras utilize continuous learning mechanisms, allowing them to adapt to new patterns over time, thereby reducing false positives and enhancing overall reliability in security monitoring. It will take at least a year for us to manage biases with more than 95% accuracy.
Q8. How did your team overcome the challenges of integrating advanced on-device AI processing, especially with the NVIDIA Jetson Nano?
Rashmi: Integrating advanced on-device AI processing with the NVIDIA Jetson Nano presented challenges due to its limited computational resources. To address these, our team employed several optimization strategies. We utilized NVIDIA’s TensorRT to accelerate inference by optimizing neural networks for the Jetson Nano’s architecture, achieving significant performance gains. Additionally, we applied model quantization and pruning techniques to reduce model size and complexity, enabling efficient execution without compromising accuracy.
We also leveraged the Jetson Nano’s GPU capabilities to parallelize tasks, enhancing processing speed. By combining these approaches, we successfully implemented sophisticated AI functionalities directly on the device, ensuring real-time performance suitable for various applications.
Q9. What measures have you taken to ensure data security and privacy, especially since the processing occurs on the device?
Rashmi: To ensure data security and privacy during on-device processing, we have implemented several robust measures. First, we employ hardware-based security features, such as Trusted Platform Modules (TPMs), to establish a secure execution environment, safeguarding against unauthorized access and tampering.
Second, all data processed and stored on the device is encrypted using advanced encryption standards, ensuring that sensitive information remains protected even if the device is compromised.
Third, we implement secure boot processes to verify the integrity of the device’s firmware and software at startup, preventing the execution of malicious code. Additionally, we regularly update our security protocols to address emerging threats and vulnerabilities, maintaining a proactive stance on data protection. By integrating these measures, we provide a secure and private environment for on-device data processing, aligning with best practices in edge AI security.
Q10. What are your current and potential revenue streams? Which aspect—hardware sales, developer fees, or subscription services—has been the most effective source of income for IndoAI?
Rashmi: IndoAI currently generates revenue through hardware sales, subscription services, and developer fees. Hardware sales involve one-time transactions for AI cameras, with pricing tiers based on specifications. Subscription services are offered via the Appization marketplace, where users pay recurring fees to access and update AI applications tailored to their needs.
Developer fees come from third-party developers who create and monetize applications on IndoAI’s platform. Among these, hardware sales have been the most successful, providing a solid foundation for the company’s growth.
To expand, IndoAI plans to enhance its subscription services by introducing more specialized applications and features, thereby increasing recurring revenue. Additionally, the company aims to foster a vibrant developer community by offering comprehensive support and incentives, encouraging the creation of innovative applications that can be monetized through the platform.