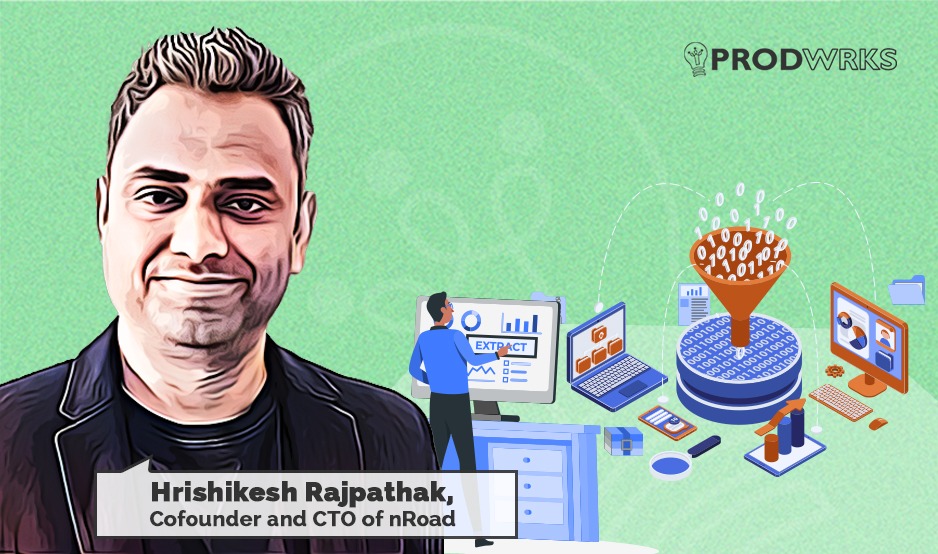
Data is used in every sector, from healthcare to finance to legal. Companies are collecting more data than ever, but extracting valuable insights from this data is a challenge. They mostly rely on manual data extraction, which can be time-consuming, expensive, and error-prone.
To address this challenge in the financial services domain, Aashish Mehta (CEO), Hrishikesh Rajpathak (CTO), and Prabhod Sunkara (COO) founded nRoad in 2021. This Massachusetts-headquartered, Pune-based company uses contextual and domain-aware AI for data extraction, helping businesses make better decisions about their products and services.
To learn more about nRoad and how it uses AI to address data extraction challenges, we interviewed Hrishikesh Rajpathak, co-founder and CTO of nRoad. Hrishikesh shared insights on nRoad’s journey, technological innovations, and the challenges and opportunities ahead.
Market Gap and Origin of nRoad
The inception of nRoad wasn’t merely a spark of inspiration. It was a response to tangible data extraction problems deeply rooted in the BFSI space.
Delving into the intricacies of the BFSI sector, Hrishikesh said, "Banks and insurance companies work with a lot of unstructured data. They work on understanding documents, extracting data from them, and then making decisions based on their extracted data. These large companies employ significant human resources to do this manually today. That's where the market gap is."
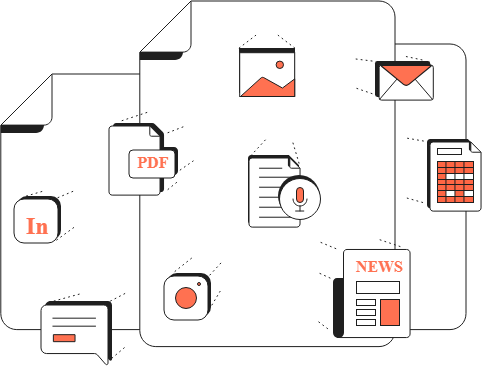
Since Ashish and Prabodh had a BFSI background, they intimately understood the issues in the sector and the multi-billion dollar opportunity for solving them using technology. With Hrishikesh’s deep expertise in artificial intelligence and data science, the trio collaborated to start nRoad and find a solution to these problems.
Hrishikesh said, "My experience was significant in natural language processing, machine learning, and deep learning algorithms. So, understanding the text, whether structured or unstructured, was one of my interest areas and expertise. We realized that introducing AI in a particular manner could give us a significant amount of delta in solving this."
With a clear problem statement and a validated idea, nRoad developed proof of concepts (POCs) to validate the product hypotheses of their AI solution.
Hrishikesh said, "The solution validation came from initial POCs that we did with large industry names in banking and rating agencies. They really loved it. Our solution did significantly better than established names that were also trying to solve this problem. Based on the performance of our POCs we were confident that we had a really good technology stack and approach to handle the problem."
Today, the POC has evolved into a next-generation AI platform – Convus, that abstracts and incorporates unstructured data and documents into vital business functions.
Role of Knowledge Graphs and LLMs in nRoad
In delving into the workings of nRoad and its product, our discussion centered on how technology enhances the entire data comprehension process. nRoad specializes in decoding unstructured data, including text in paragraphs and data presented in tables.
Hrishikesh commented, "Large Language Models (LLMs) are the primary tool in our tech. These LLMs serve a dual purpose. For paragraph data, they contribute to the creation of knowledge graphs, a key focus of nRoad's analytical approach. Simultaneously, the team has devised a proprietary method for tabular data analysis, connecting it seamlessly with knowledge graphs derived from textual data."
A knowledge graph is typically a format for representing knowledge taken from any source. While relational databases capture data, knowledge graphs transcend by encapsulating not just raw data but also the associated information and insights that elevate it to knowledge. This involves understanding not only individual data points but also their interconnectedness.

Hrishikesh added that the connection between different data parts or data sources and creating a singular picture out of the entire document or entire source of data is made using LLMs.
"But again, it's not just the LLMs that we use; we use LLMs and other technologies to create this knowledge representation."
In their quest to optimize this process at nRoad, Hrishikesh found that experimenting with multiple ways of handling this problem, “a combination of ontology + LLMs + knowledge graph,” brings a lot of value to us in solving this problem.
Beyond LLMs - Multifaceted Tech Stack of nRoad
While LLMs are pivotal in accelerating the processes at nRoad, they are just one component of the comprehensive technological toolkit.
Hrishikesh said, "LLMs are one big part of our tech. Then, there is a knowledge presentation and knowledge graphs. There are some vision-based deep learning techniques that we use for pre-processing and extraction of data."
Hrishikesh also commented on the workflow, which typically involves the initial extraction of data, its conversion into a specific representation involving knowledge graphs and databases, and finally, the data analysis phase.

"In the data analysis phase, the core LLMs and other NLP algorithms come into the picture. Data extraction has vision-based deep learning algorithms that we use. It's a different machine learning and deep learning model that are significantly dependent on vision-based algorithms."
Hrishikesh adds, "The subsequent stages encompass storage and representation, where databases and knowledge graphs come into play, followed by the analytical phase, where LLMs, other Natural Language Processing (NLP) algorithms, and various machine learning algorithms synergize to provide a comprehensive solution."
nRoad's USP - Context Sensitivity and Domain Awareness
The assertion that nRoad’s extraction algorithm is trained to be context-sensitive and domain-aware is a strategic necessity deeply ingrained in solving enterprise problems.
Hrishikesh explained, "We cannot treat a document as just an English language document. There is a lot of domain context that comes into the picture. The critical concepts in that specific industry differ. So, when analyzing data, we cannot say that our algorithm should be able to understand English better or different languages better. We also brought domain knowledge into our models."

The challenge lies in infusing this domain knowledge into the models effectively. To overcome this hurdle, nRoad employs a multifaceted approach.
Hrishikesh explains, "The models we created have dual layers - one that comprehends the universal language and another fine-tuned to grasp the nuances of a specific domain. This nuanced layering ensures that the machine learning and deep learning models don't merely process language; they become attuned to the industry's distinctive intricacies, making nRoad not just context-sensitive but inherently domain-aware."
This approach adds a layer of sophistication to nRoad’s capabilities, elevating it to a level where it doesn’t just read data; it comprehends the context based on the industry or domain.
Product Evolution and User Feedback Integration
On addressing the intricacies of user feedback collection and its integration into the product roadmap, Hrishikesh reveals a comprehensive approach adopted by nRoad.
Hrishikesh explains, "We have the whole product built in a manner where everything the user does on that product goes back to the machine learning algorithms. There's continuous training that happens. So, when users review and modify data within the platform, this feedback seamlessly integrates into the evolving models, progressively enhancing their accuracy and efficacy."

In response to queries about the nature of data collected, Hrishikesh elucidated that, unlike traditional surveys, nRoad relies on a distinctive approach, presenting machine-generated outputs to users.
"Leveraging the concept of Reinforcement Learning from Human Feedback (RLHF), end users are empowered to review and modify the presented data before final acceptance."
This human-in-the-loop model ensures that qualitative aspects are thoroughly examined in sectors demanding thorough examination, such as banking and finance.
"Now that output, whether qualitative or quantitative, goes back to the machine. For the next iteration, the machine would have learned that a similar case was defined by a human in this manner. So next time, whenever it gives a similar kind of output, it'll integrate that feedback and ensure that continuous learning keeps happening."
The RLHF framework not only caters to quantitative adjustments but significantly emphasizes the qualitative dimension, fostering a continuous learning loop where human insights inform and enrich machine learning models.
Key Product Metrics - Extraction Accuracy and Analysis Accuracy
In shedding light on key product metrics and analytics monitored on the nRoad platform, Hrishikesh underscored the importance of data accuracy, extraction accuracy, and extraction completeness.
"But many metrics come from clients since we work with enterprises. So, different clients have different ways. Based on their problem statement, they decide what they wish to measure, and we build those in our metrics accordingly."

This bespoke approach ensures a tailored and effective measurement framework aligned with the intricacies of individual client needs.
Delving into the nRoad’s north star metric, Hrishikesh reveals that extraction accuracy and analysis accuracy, collectively termed ‘normalization,’ stand out as the north star metrics. These metrics serve as the bedrock of nRoad’s product evaluation, reflecting the industry standards and the essence of the product’s efficacy.
Challenges in Data Curation and Technological Evolution
This transformative journey is not without its hurdles, and the Hrishikesh sheds light on the multifaceted challenges faced by nRoad.
Hrishikesh states, "Since machine learning is data-dependent, there are data challenges. We have to make sure that we curate the data correctly. That's why we employ many domain experts, not just technical people. These experts ensure that the data curated for model training is not only sanitized and clean but also impeccably represents the intricacies of the industry."
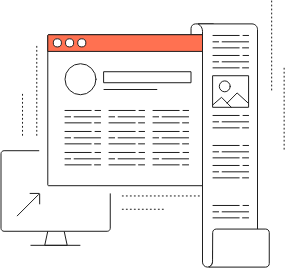
According to Hrishikesh, the challenge lies in striking the right balance – capturing a broad industry spectrum without introducing biases.
"That's where our domain experts come into the picture, and we work with them to ensure that the models we create are well-rounded and good enough to understand these things," said Hrishikesh.
Technology’s relentless evolution constitutes another challenge. Hrishikesh expressed that continuous improvement and rolling out of newer stock is another challenge.
"As technology keeps evolving, we must constantly keep refining, introducing newer concepts at times, even wholly taking away what we have done and altogether introducing something new and, then redoing, training it again for that particular domain."
Moreover, the ever-pressing need for quick turnaround times adds another layer of complexity. Hrishikesh emphasizes building technology that not only meets the rigorous demands of continuous research and development but also adeptly navigates time constraints.
In essence, nRoad confronts and conquers these challenges through a harmonious blend of data expertise, technological agility, and unwavering commitment to advancement.
nRoad's Vision for the Future - Search-based Algorithms
Our interview with Hrishikesh sheds light on nRoad’s future trajectory, outlining plans to introduce cutting-edge methodologies in data representation, knowledge representation, and knowledge search.
Hrishikesh foresees, "In the future, from an AI point of view, search-based AI algorithms will be equally disruptive as LLMs. So, we are also doing a lot of research on that. We have had some interesting insights and some exciting breakthroughs on that front. In the coming months, we will see a lot of new technology from nRoad on that front."
In our exchange, Hrishikesh also addressed the pervasive discussions surrounding LLMs on social media. He emphasizes a notable gap in the ongoing discussions where people are oversimplifying by attempting to rely exclusively on LLMs for comprehensive problem-solving.
"LLMs have a vast potential to change how we approach the problems. So, people have started exploring this particular technology. In my opinion, LLMs are going to basically augment the entire process, but not going to solve the whole problem."
For organizations venturing into AI adoption, Hrishikesh cautions against relying on a singular solution to address complex challenges. Instead, he advises organizations to embrace a strategic amalgamation of various technological advancements.
Drawing from practical experience, Hrishikesh explains, “We have already fine-tuned and developed our own underlying open-source and LLM models. It has made things much easier, but it’s not the only thing that can be used to solve the problem completely.”
He advises, "LLMs might significantly change how you do things. Still, it is essential at the same time to experiment with those LLMs, do research, find out what you can do to add more value to the LLMs, and how you can fine-tune those LLMs better for your problem statement."
Hrishikesh says that this multifaceted approach, combining industry trends with internal research, is a critical strategy for achieving success in AI adoption.